Q&A Part I with Dr. Wendy Tate, Author of Insights Accrual Prediction Algorithm
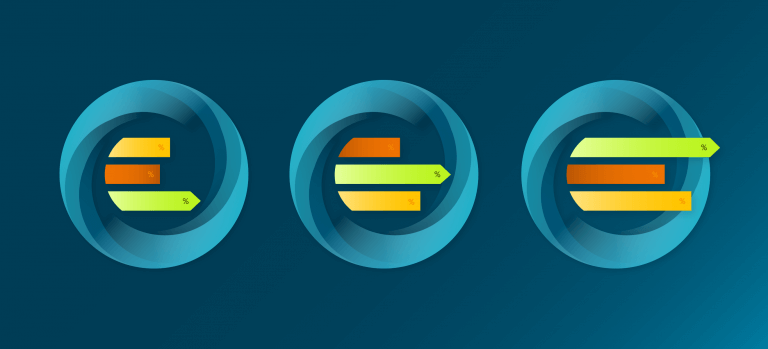
In every aspect of her research career, Dr. Wendy Tate, Director of Research Operations at Advarra, has been committed to efficient, effective research. With a background spanning laboratory, regulatory, and oncology, Wendy has experienced firsthand the challenges in accurately predicting accrual at a research organization. In 2014, spurred to improve clinical operations, she set out to develop a better method to predict accrual. Last week, Advarra launched a pilot of the Insights Accrual Prediction platform to customers, powered by Wendy’s initial model and collaboration with Advarra’s Onsemble Community. Presented in two parts, we sat down with her to learn more about the inspiration for leveraging machine learning technology in the research industry, how the model came to be, and what she envisions for its future.
Let’s start at the beginning. When did you first gain interest in predictive modeling and realize its potential to be applied in clinical research?
Wendy: I first realized the potential of predictive modeling while in the Pharmaceutical Economics, Policy & Outcomes PhD program at the University of Arizona. Several classes within the program focused on using data to investigate how outcomes can be measured, how medications are managed, and using data to drive treatment and care. During that program, I left my position at the IRB and joined the University of Arizona Cancer Center clinical trials office. As the assistant director overseeing the administration of that office, one area of focus was to ensure that our clinical trials were meeting accrual goals. This allowed me to gain insight into how accrual feasibility decisions were made. Eventually my medical director and I asked ourselves, “why aren’t we modeling accrual?” and so began the desire to take what we were learning in our respective graduate programs and bring it to the clinical trial office to improve our research. We wanted to bring objective data and empirical evidence to our efforts so we could better use our staff and funds, and help our investigators conduct more successful research.
Why do you think the clinical research industry, while innovative, is wary to adopt more cutting-edge technology and processes?
I think there are quite a few bases we need to connect. First, when people get into research, they are more interested in innovation from the lens of medicine, treatments, biological, physical, and chemical pathways than the infrastructure that those innovations must pass through for approval. The focus, as it should be in medicine, is the patient; curing disease, bettering public health, improving global outcomes. If a process is working, even sub-optimally, it is often still viewed as better than disrupting an invention that could bring a new treatment to market.
The field of people researching how to conduct clinical research is very small. A lot of the frameworks that I based my work on are coming from the industrial organization and management area. Because our industry is geared very much toward treatment, we have a hard time viewing ourselves and our work as a business that needs to scrutinize our processes for improvement. However, in order to maximize benefit to patients, we must recognize that ‘no’ is sometimes the best answer. That is a difficult position for many research leaders to take. Practitioners and clinical investigators want to treat; they want to see good science get to the people who need it. I would argue that if you cannot keep a clinical research program solvent and staffed, you will help no one because you will not be able to conduct ethical and compliant research.
There is some truly excellent research leadership across the nation. However, we often see research leadership promoted into management from a scientific position, which results in limited training (or maybe even desire) to assess clinical research as a business. Those in the business community don’t often realize the opportunities for their skill sets and training in the clinical research sector. We’re starting to see a shift across our Onsemble Community and centralized clinical trials offices, hiring more MBAs and getting more scientific staff business training. As organizations begin to prioritize business strategy, improve communication with investigators on scientific priorities, and leverage tools such as analytics and process analyses, the clinical research industry will evolve like we’ve seen with other cutting-edge technology industries.
How has your data-driven approach helped you in a clinical research setting, and why do you think the Accrual Prediction Platform is exciting for our community?
Investigators are incredibly optimistic people whose first priority is to help patients. I’ve seen this especially in oncology—they are helping people take on, and in many cases beat, one of the worst diseases imaginable! They are dedicated practitioners who are devoted to their patients and science. One of the problems running a CTO with a group of optimists is that they think they can open a lot of trials and enroll a lot of people onto all of them. While I as a scientist would also love to do that, I as an administrator know that we have limited staff, clinic space, institutional funds, etc. That’s where data helps to open those lines of communication. Data shouldn’t make the decision for you, but it should support your conversation. The conversation allows you to create a plan of attack. Should we open this trial? Should we revise the original proposal? Should we involve other staff or satellite clinics? The answer doesn’t always need to be ‘yes’ or ‘no’ but instead, ‘if yes, what resources does it need to succeed?” Or “Not right now, but after another study is finished.” This helps everyone be more successful.
What has been the response to your ideas along the way? Who was excited, who was skeptical?
There will always be dissenters to any new change. From initial pushback on the dissertation idea to introducing it to investigators, no one wanted to hear ‘no’ or to have their leadership trust a machine more than their experience and expertise. But clinical trials are getting more complex with increasing amounts of exclusion and inclusion requirements. We want to help the investigator understand which factors may influence their success. I keep going back to the conversation element, but really that’s what we’re trying to support.
Moving forward, our goal is to talk to our pilot customers, to explore different feasibility information applied to diverse communities and situations to ask, “Does this help you?” and “If so, how?” One of the most interesting elements is researching the model itself. We’re working closely with our pilot customers to explain how this model works and what informs the output, so when they have conversations with their investigators, everyone involved knows what is supporting this discussion. They can explore which organizational factors appear to influence their ability to accrue to protocols. This can help guide process improvements, identify roadblocks, and ideally improve their overall research operations by better understanding themselves.
Check back tomorrow to read the second installment of our conversation with Wendy, where we’ll dive into the arguments for and against predictive modeling, and discuss how this technology could be leveraged in the future.